4:0min
Having trained as a computer scientist, Andrew Turpin didn’t begin his career with an expectation that he would end up in optometry, but after working on visual field testing as part of his post doctorate he found his passion for computer science matched up well with practical applications in eye testing.
What is intelligence? Is it the ability to hold a conversation and retrieve information, like modern creations such as ChatGPT are capable of, or does it require more complex interactions of billions of neurons present in the human brain?
Andrew Turpin has encountered these questions and more throughout his career. After he completed a PhD in data compression at The University of Melbourne, he sought to expand his understanding of computer science with a post doctorate at the Devers Eye Institute in Portland, Oregon. Here he met a psychophysicist named Chris Johnson, who was engaged in studying new ways of testing human vision without medical intervention. This involved showing people novel visual stimuli and recording what happens to their perception when they have eye disease.
This area of research intrigued Andrew. Visual field testing can interact with other senses, such as creating an audio illusion through visual stimuli, and can be useful for testing people’s vision, colour range, and more. It wasn’t long before Andrew found himself working with visual field algorithms to enhance this process.
Now working with visual field technology in Perth, Andrew will be the keynote speaker at Optometry Virtually Connected in June, where he will speak about AI and data in eye care. Andrew’s keynote address will cover two things: in practical terms, he will explain the uses and limitations of machine learning and deep learning when it comes to analysing eyes, looking behind the eye, and what to watch out for with this technology. But before he can explain that, he will cover the key differences between “artificial intelligence” (AI) and “machine learning”.
AI and machine learning: what’s the difference?
While these terms are now used interchangeably, they actually mean two different things. “Machine learning” defines a piece of software that makes decisions based on the data it’s given by finding and learning patterns within the data. For instance, it can learn what a diseased eye looks like from existing data, and then use that pattern recognition to analyse new data – a new eye – and give a probability of a certain outcome.
‘The reason it’s called “learning” is because we do that too. A lot of our “fast thinking” is pattern recognition, like recognising stop signs or riding a bike; we’ve seen or experienced lots of examples over our lifetime and we’ve incorporated that into our lives. But machine learning can’t make deductions, and it can’t do deeper reasoning. It’s all just based on the data you give it. If you give it insufficient or incomplete data, it won’t work well. The idea of “garbage in, garbage out”,’ Andrew explained.
When it comes to eye care, this can be a problem. If you’re teaching a system to find diabetes, and you only give it examples from one demographic, such as advanced age, then try to apply that learning to another demographic, like younger eyes, machine learning won’t make correct predictions. It’s only as powerful and useful as the volume and range of data you feed it.
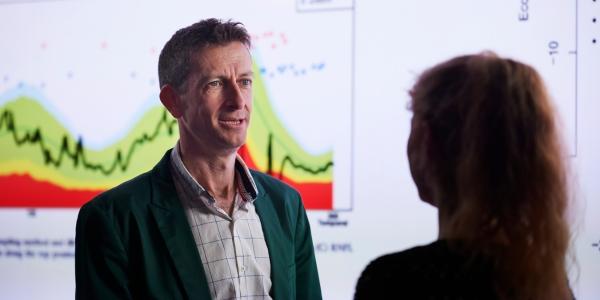
All current “AI”, including the much-lauded ChatGPT, is just advanced machine learning provided with access to a large volume of data. But Andrew explains that using ChatGPT in a practical setting, such as using it to explain things to patients, could be dangerous.
‘ChatGPT seems intelligent at first, as it gives sensible answers, but, to quote computer scientist Jason Lanier, just because software looks more flexible and accurate than previous software doesn’t make it intelligent. It’s certainly an amazing piece of engineering, particularly for people who don’t have English as a first language or those who need to produce procedural text. In an optometry context, this could mean things like referral letters to doctors or marketing materials. But it’s not particularly useful for scientific purposes, as it doesn’t necessarily tell the truth.
‘It’s not “intelligent” in the sense of human intelligence. If you ask it to solve a Sudoku, for instance, it will just return a random result not based on logic. If you however ask it to write a program to solve sudoku, it can do that, because then it’s back to its strength and purpose – pattern matching,’ said Andrew.
So what is true artificial intelligence, as opposed to machine learning? According to Andrew, it would need to be a machine or program that exhibits independent intelligent behaviour. But what constitutes intelligence?
‘That’s a philosophical question, really. I suppose part of it would involve reasoning, such as being able to justify why you’ve given a particular answer, and then there’s the ability to undertake abstract thought. These are things you don’t see in most current machine learning or “AI”.
‘That said, machine learning algorithms like those used in ChatGPT are what we call “Deep Learning”, where the inner workings are so complex that they can’t be explained or traced back in a way a human can understand. For instance, if it gets a sudoku wrong, it will still insist it is correct, despite it never having been programmed to lie. It’s not sentient, it has no body or job or mortgage, it has no reason at all to lie, so in a sense it’s not even really lying. But it’s also not telling the truth either,’ he said.
Measuring intelligence
Andrew explains that ChatGPT has about 175 billion ‘parameters’, each of which constitutes a node of decision making conceptually analogous to a neuron in a human brain. When ChatGPT produces an answer, it is beyond human comprehension to understand what combination of parameters among those 175 billion have been drawn on to generate that answer. And if you asked ChatGPT, it wouldn’t necessarily even give you a correct answer, because the program doesn’t know itself.
‘For reference, a human brain has six hundred trillion synapses, so we’re about one six-hundredth of the way to the complexity of the human brain with machine learning programs like ChatGPT,’ he said.
And then there’s the limiting factor of input data – for each parameter, you ideally need at least one data sample, so even if scientists could create a machine learning algorithm with the full complexity of a human brain, we may not be able to scrape together enough data to train it. Andrew explains that the internet has roughly one hundred trillion words on it, much of which is duplicate, so even downloading the entire internet wouldn’t come close.
‘To quote the Australian AI professor Toby Walsh, the problem isn’t that the machines are too smart, it’s that they’re too stupid,’ he said.
How do humans utilise our six hundred trillion neurons of brainpower? By using our senses.
‘If you think about how much data we get from our senses, the numbers stack up pretty quickly. Say we’re sampling 50 megabytes of image data a second through our eyes. That’s 3 gigabytes a minute, 180 gigabytes an hour, 1.8 terabytes of data a day, just through just our eyes. That doesn’t count ears and other senses, all of which are constantly collecting data and cross-referencing with each other. Our neural network is trained on vast amounts of data every day throughout our lives to inform our decision making, and no machine learning that currently exists can match that.
‘Maybe over the next 10 years, it would be feasible to build a human-brain level network with a camera, a microphone, pain sensors, and so on, and in theory that would be enough to create intelligence, although it would be the equivalent of a newborn, so you’d have to let it run for 20 years to approximate an adult. It would have to learn to run, eat, kick a footy, and all that goes into forming human intelligence,’ Andrew explained.
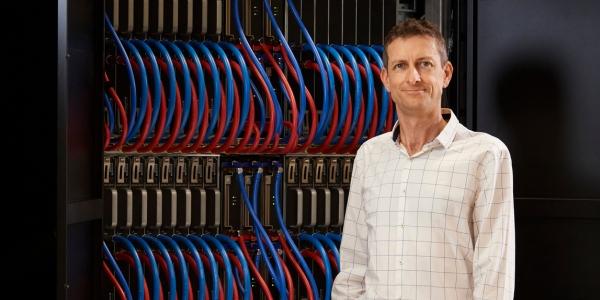
The science is far from settled – we as humans still don’t truly understand what “consciousness” is. In theory, if you create a machine with enough neurons and feed it enough data, and there’s not some special quality about the brain we don’t understand, then you could create genuine artificial intelligence. While it hasn’t happened yet, Andrew shares that current models have reached one trillion parameters, so it’s only a matter of time before we get closer to finding out for sure.
Applying machine learning to optometry
So where does optometry come in?
‘Lots of people are doing things with machine learning and deep learning, but it’s not obvious to me it’s that useful. Most of it is smart engineering with no current practical application. I’m interested in doing work with AI that’s useful, and my interest is more in an applied health-system point of view, like what kind of AI would be worthwhile in a medical situation, or in this context, for optometry,’ Andrew explained.
In practical terms, optometry will likely see the introduction of tools based on machine learning that can be used in the practice, particularly when it comes to imaging. A well-trained machine’s ability to recognise patterns can’t be matched by a human, particularly if time is a factor, so this could speed up results of imaging significantly. Home monitoring may also see significant change, with algorithms that can monitor for and accurately understand user-submitted data. But machine learning can also be useful on the less technical side of running a practice. Andrew foresees a ChatGPT-like tool that can talk to patients and manage automatic telehealth triage, although he admits that may be a way off yet.
‘There’s a lot of techno-optimism. We can build systems that have high accuracy in a controlled environment, but as soon as you deploy them in the real world, like a busy optometry practice where the data is noisy and unexpected, they don’t work as well,’ he said.
Whether or not the new tools will be relevant in a real-world setting remains to be seen. For Andrew, the technology itself won’t be the barrier for adoption. Data-confidence will improve, the ability to explain conclusions will improve, and training-bias in the data will be amended. Instead, it will be the social and political arenas where the future of machine learning will play out most conspicuously.
‘People need to believe in these tools before they get adopted. The power of the technology, whether it’s currently more or less accurate, whether or not it reveals more truth in data than we can see ourselves, that’s all far less relevant than whether people are willing to accept it or not. The social factors will matter more for adoption than the technological ones,’ he said.
Andrew will be the keynote speaker at Optometry Virtually Connected on June 17-18.
Tagged as: AI, andrew turpin, machine learning, Optometry Virtually Connected, OVC